HOOT is geared towards training, evaluation and analysis of algorithms more robust to occlusions. HOOT annotations include extensive frame-level occlusion labels, including occlusion masks!
We provide a training and test split for development, or you can use the entire dataset!
500+ High-Occlusion Videos
High-Quality videos of every-day objects for single-target tracking under heavy occlusion (median of 68% of frames occluded per-video).
HOOT will grow! Please follow this page for updates to the dataset.
Extensive Occlusion Labels
Each frame is densely-annotated by absence, full occlusion, cut-by-frame, partial occlusion by object attributes and more!
All 400K+ bounding boxes come with occlusions masks!
Defined Occluder Types
Occluder types defined (e.g. solid vs. transparent) and frame-wise masks are given for each type.
Types hint at how much the occluder might be affecting object appearance, without expensive pixel-level annotations.
Announcements
- 01/05/2023 - We presented the HOOT Benchmark as part of WACV 2023, in Waikoloa, Hawaii. [Presentation Video] [Bibtex]
- 12/30/2022 - HOOT website and toolkit are live! [Licensing Info] [HOOT Toolkit]
Our Team
Authors
Gozde Sahin
PhD Student
University of Southern California
Los Angeles
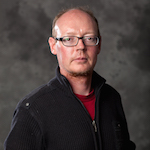
Laurent Itti
Professor of Computer Science
University of Southern California
Los Angeles
Annotation Team
MSc Students in University of Southern California (USC)
Sampath Mangalapalli | Sai Yoshitha Akunuri | Aishwarya Sitharam | Vinya Somayajula |
Paridhi Shah | Ketaki Lolage | Vimal Shah | Yathin Kumar |
Xinyue Yu | Yunhao Han | Manav Jain |
Contributors
Ayush Jaiswal | Fatma Sahin | Amanda Rios | Ankit N. Bhawsar |
Toy Leksut | Ruitong Sun | Beril Erkin | Harrison Lee |
Burcu Yeni | Aysu Akay | Tamer Sahin | Yunhao Ge |